Reinforcement Learning 2:0
Prof. Shalabh Bhatnagar
Department of Computer Science and Automatio, IISc
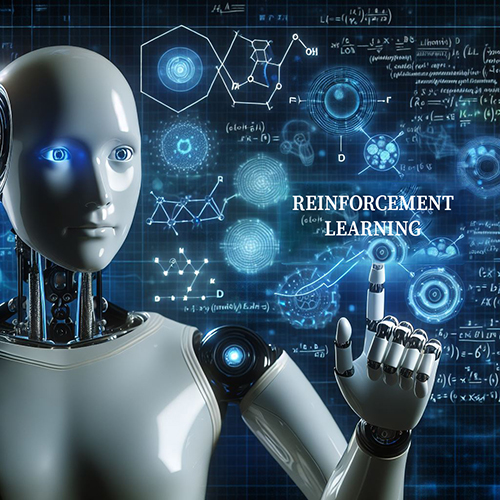
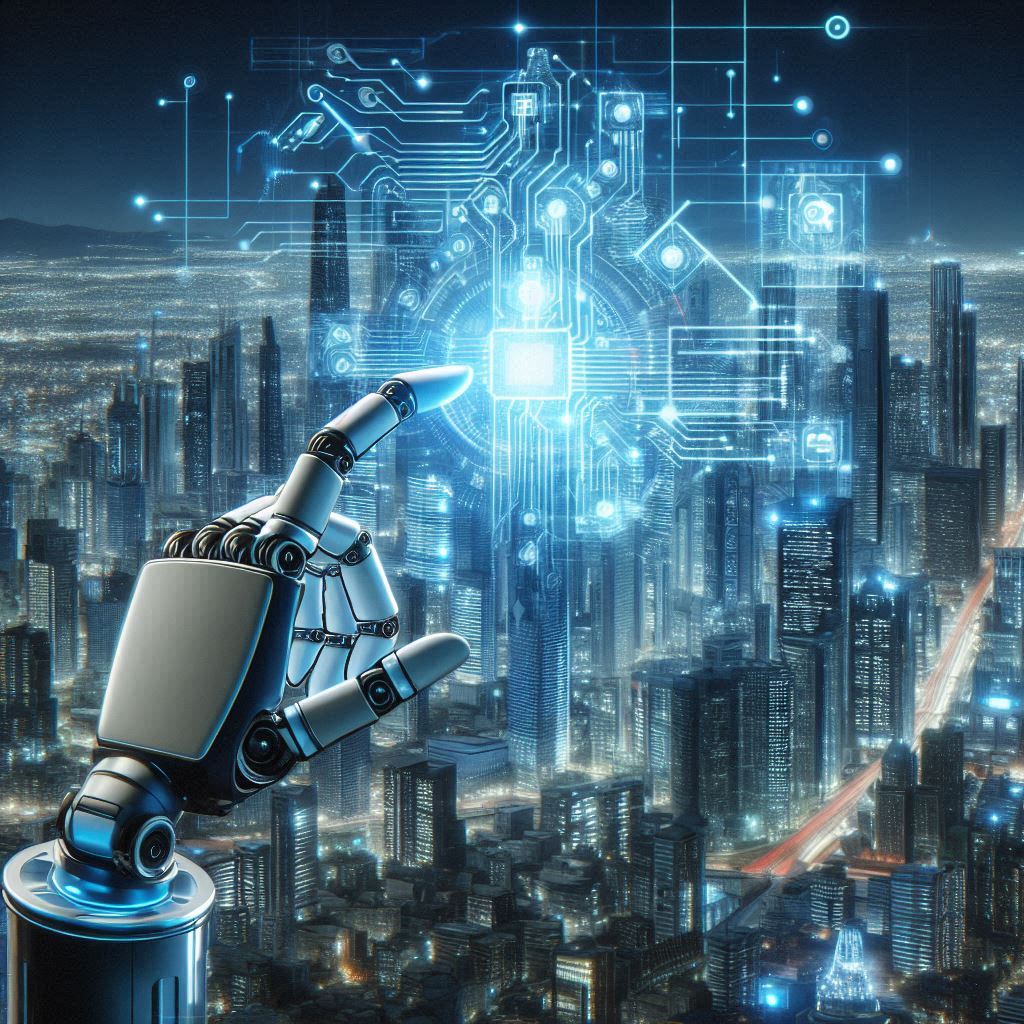
Objective of the course
The objective of the course will be to provide both a rigorous foundation in Reinforcement Learning through the various tools, techniques and algorithms used as well as cover some state-of-the-art algorithms in Deep Reinforcement Learning involving simulation-based neural network methods.
Syllabus
Introduction to Reinforcement Learning, Multi-armed bandits, Markov decision processes, Dynamic Programming – Value and Policy Iteration Methods, Model-Free Learning Approaches, Monte-Carlo Methods, Temporal Difference Learning, Q-learning, SARSA, Double Q-learning, Value Function Approximation Methods – TD Learning with Linear Function Approximation, Neural Network Architectures, Deep Q-Network Algorithm, Policy Gradient Methods, Actor-Critic Algorithms.
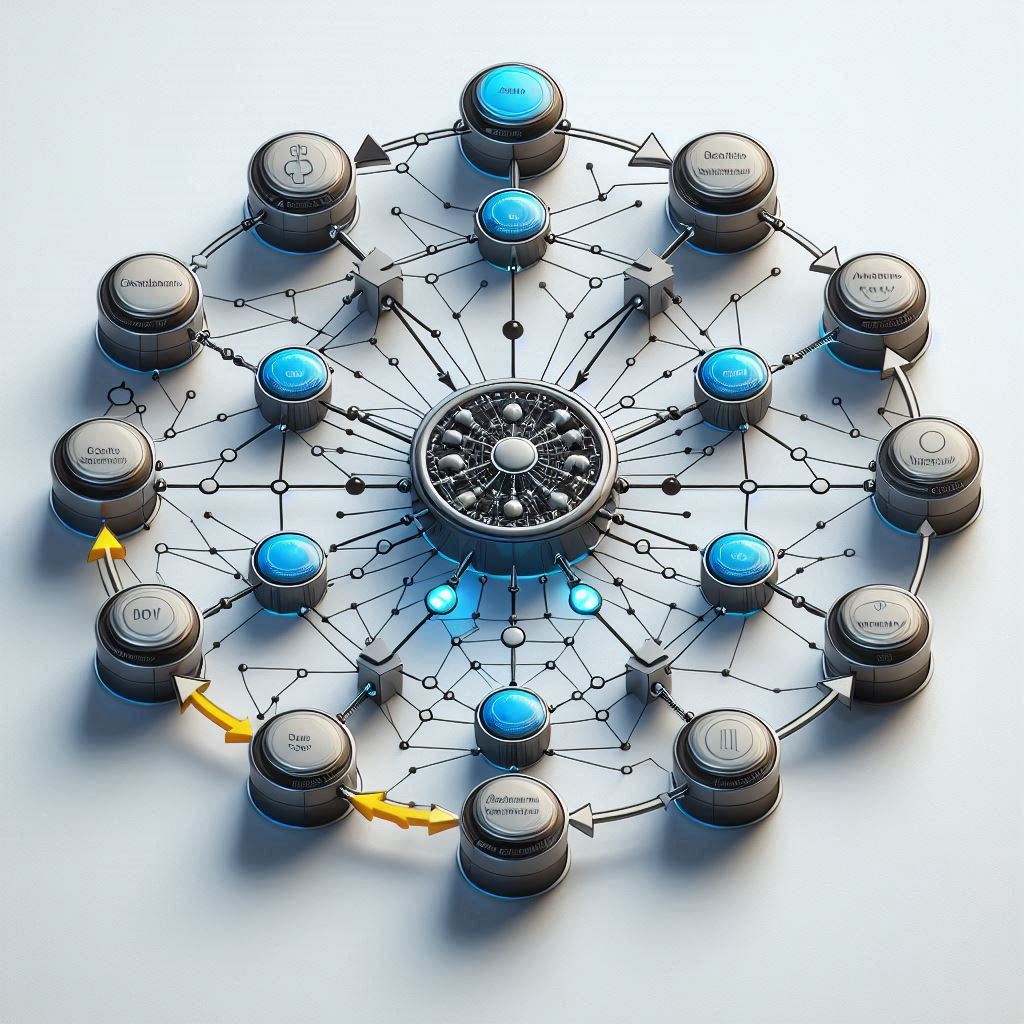
WEEK Topic to be covered
Week 1: Introduction to RL
Week 2: Multi-armed bandits
Week 3: Markov decision processes
Week 4: Markov decision processes and dynamic programming
Week 5: Dynamic programming algorithms
Week 6: Monte-Carlo algorithms
Week 7: Temporal Difference Learning: the full-state case
Week 8: Temporal Difference Learning: full state algorithms
Week 9: RL with linear function aproximation
Week 10: RL with nonlinear function approximation: Deep Q-Networks
Week 11: Policy gradient methods
Week 12: Policy gradient methods
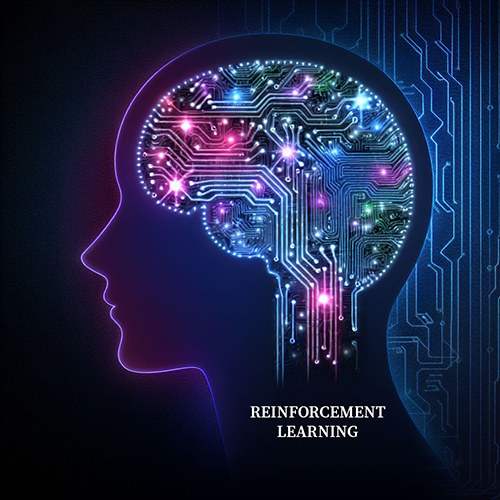
Minimum Qualification
B.Tech in any discipline or B.Sc/BA in Physics/Chemistry/Maths/Statistics/Computer Science/Economics
Pre-requisites
NIL
Who can apply?
Any person from the industry or academic institutions should benefit.
Number of credits – 2:0
Mode of Instruction:
Online with Synchronous mode
Online Seats are Limited to 100
Last date to apply:
30 April 2025
Duration:
1 May to 31 July 2025
Timings of the class:
Tuesday & Thursday 8:30 P.M. to 10 P.M.
Exams:
25 – 31 JULY, 2025
Course Fee
Particulars | Amount (in ₹) |
Course Fee | 10,000 |
Application Fee | 300 |
GST@18% | 1,854 |
Total | 12,154 |